Is basketball more entertaining than football? Is an SUV a better vehicle than a sedan? Is it healthier to eat spinach or eggs? A natural response to questions like these would be, “Can’t say…you can’t compare apples and oranges.” However, we still could safely offer our opinions without misleading anyone in a harmful way. But not so with educational researchers, who have the serious responsibility of reporting credible results regarding the efficacy of interventions. Credible results come from rigorous research studies.
Rigorous studies that meet “moderate” and “strong” evidence standards for the Every Student Succeeds Act (ESSA) and the What Works Clearinghouse must compare relevant and objectively measured educational outcomes, such as student achievement or graduation rates, for the intervention group and an “equivalent” counterfactual (or control) group. For both logical and scientific reasons, comparing an apple to an orange, such as a high-achieving intervention group to a struggling control group, won’t pass the evidence taste test.
Randomized-controlled trials (RCTs), while more challenging than quasi-experimental designs (QEDs) to implement in school districts, offer an important advantage for obtaining equivalent comparison (apples-to-apples) samples in experimental studies. By giving every study participant (student, class, or school) an equal chance of being assigned to the intervention or comparison group, RCTs thereby eliminate any systematic selection bias and have a very high probability of achieving sampling equivalence. QEDs, while easier logistically to implement, do not offer the same protections. In a typical QED, participants decide whether to adopt the intervention based on interest to continue using the existing (business-as-usual) program. Consequently, there is risk that these two groups intrinsically differ in background, skills, and educational environments (school quality, resources, peer support, etc.). Recognizing these risks, the WWC and Evidence for ESSA both require that the intervention and comparison samples do not differ by more than .25 standard deviations at pretesting.
Recently, we were asked by a program developer to evaluate a literacy intervention that only the Title I schools in the school district were implementing. None of the potential comparison schools was Title I and all were higher performing than the intervention schools. Could a credible study be performed? The definitive answer is “maybe,” but the results might be suggestive at best. As we describe below, there are strategies for making oranges more like apples.
One popularly used strategy is propensity-score weighting (PSW; Morgan & Winship, 2015). Here, the analyst statistically weights cases based on the probability of an observation (i.e., student) being in the treatment group. While several weighting methods exist, the rationale behind each weighting method is to use regression methods to estimate for each student how likely it is for them to be in the treatment group, on the basis of variables that usually include prior achievement and demographics. From our example above, if Title I students were more likely to be in the program, then you would want to focus on (and weight more) the Title I students from the comparison group. The result is that comparison students who are most similar to treatment students (on the basis of variables used in the weighting process) are weighted more heavily in analyses, while students that are less similar to treatment students are weighted less. Over the whole sample, this results in a comparison group that looks more similar to the treatment group, making it more likely that treatment and comparison conditions are baseline equivalent.
Another commonly used strategy is propensity-score matching (PSM; Morgan & Winship, 2015). In this approach, the analyst matches treatment students to one (or more) comparison students, again, on the basis of prior achievement and demographic variables. There are many different matching approaches to use to determine the best comparison student match (or matches) for each treatment student. As with propensity-score weighting, the end result of this process is a comparison group that is more similar to the treatment group in terms of prior achievement and demographic variables.
However, there are limits and logical guidelines for when such sampling adjustments can be applied and when the differences are just too great for the study to yield trustworthy evidence. Here are our suggestions:
- The intervention and comparison samples, even if similar in prior achievement, should also be comparable in demographics. For example, a comparison sample with similar prior achievement but a significantly larger percentage of special education students could potentially still raise serious concerns about sample equivalence.
- Related to this, the more demographic and background information you have on students that you can adjust for, the better your matching will be. The more lingering differences between the groups that you cannot account for, the more likely you are to have bias and error in your impact estimates.
- While large sample sizes are not required to perform PSW or PSM, larger sample sizes will allow for more statistical adjustment to be made.
- More broadly, it is important to remember that the statistical adjustments we discuss here are just that: adjustments. These methods are not meant to reconcile differences between fundamentally different intervention and comparison samples.
QEDs in educational research often involve apples to oranges comparisons, with intervention and comparison samples differing on some variables. However, the strategies discussed here can help to make the oranges into fruits that might not exactly be apples, but which are much closer to apples than oranges (say, pears), such that credible comparisons may be conducted. However, if intervention and comparison samples are fundamentally completely different (let’s call that an apples to carrots comparison), it is much less likely that these strategies will successfully transform the carrots into a food that is similar enough to an apple to yield a valid comparison.
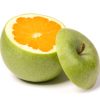