A blog from the Center for Research and Reform in Education at Johns Hopkins University
In serving as third-party evaluators of educational interventions, our center commonly hears strong preferences from our clients (product developers and school districts) for randomized controlled trials (RCT) to test program efficacy. After all, over two decades ago, the No Child Left Behind Act of 2001 (US Congress, 2001) emphasized randomized experiments as the “gold standard” for promoting scientifically-based educational research. RCTs randomly assign study participants (e.g., students or teachers) to treatment groups that will either use or not use the target intervention, thus eliminating sampling bias. This exalted position of RCTs continues and is even accentuated today by the Every Student Succeeds Act (ESSA, 2015), which restricts expenditures of federal funds to educational programs and services that show evidence of effectiveness. ESSA established four ordered tiers of evidence. At the top of the hierarchy is “Strong Evidence” (Tier 1), which requires significant positive results from a rigorous RCT. “Moderate Evidence” (Tier 2) is obtained from a rigorous quasi-experimental design (QED), where treatment groups are formed using an uncontrolled process, such as schools self-determining whether to use or not use the target intervention. Lower tiers are “Promising Evidence” (Tier 3) obtained from a correlational-type study, and a research-based “Rationale” (Tier 4) supported by a logic model or white paper. The What Works Clearinghouse (WWC), in turn, requires evidence from an RCT study to meet standards “without reservations,” whereas evidence from a well-designed QED can meet standards “with reservations.”
In the highly competitive, ever-expanding marketplace for ed-tech and other educational interventions, reaching the gold standard naturally has high appeal to industry providers as the largest trophy on the evidence mantlepiece. But, in many typical circumstances, could this quest for gold eventuate, due largely to the conditions of schools’ product adoption, in “fools’ gold”— “null effects” for the target intervention? Based on our center’s experiences in conducting literally hundreds of such evaluations, we view this risk as real and therefore requiring careful analysis of participation and implementation conditions in choosing a research design. For both evidence seekers and users, I believe that a useful question to consider is: How do the requirements and characteristics of RCTs vs. QEDs impact the potential of obtaining supportive evidence for this particular intervention in this application setting?
Being welcome in the schoolhouse. An efficacy study first and foremost requires a school district or school willing to house it. By elevating the role of evidence in program procurement, ESSA requirements have greatly expanded researcher requests for school districts to approve their studies. Today, in the aftermath of Covid disruptions, school districts are acutely stressed with addressing resultant student achievement deficits and staff shortages. Clearly, accommodating an RCT creates a much bigger “ask” than does a QED by requiring teachers/schools that are interested in the intervention to put their plans on hold (and in jeopardy) until the random drawing is completed. Not being selected likely means having to continue to use, for a year or even longer, the very programs being considered for replacement. A QED, in contrast, essentially allows schools to implement the programs that they would normally choose (the intervention or existing one), surely a more palatable option for participating in research.
Program selection as intervention attribute. As my colleagues and I found in a recent study of school districts’ procurement of ed-tech products (Morrison, Ross, & Cheung, 2019), educational programs typically are selected by school districts and schools based on some formal vetting process, some more systematic and inclusive than others. In discussing with teachers and administrators which programs prove most effective and sustainable in their schools, we frequently heard as key factors the amount of program “buy-in” by teachers and the program’s responsiveness to the school’s priority needs. In this sense, most of the efficacy studies we conduct emanate from a natural QED situation in which certain schools decide on their own (through routine vetting and selection processes) to adopt the target intervention, while others in the same district choose to stay with their existing program. Although we have had schools agree to be subject to random assignment in support of an RCT, I can recall no instance where randomization was a natural occurrence or any school’s preference for determining program usage. It therefore seems fair to raise the question whether the nature of program selection becomes an effectual and meaningful part of the “intervention” being evaluated. If so, QEDs much more than RCTs represent the way that the vast majority of schools actually will select and implement the intervention and comparison programs being researched.
Effects on effects. Given the many interventions and uncontrollable variables in real-life school contexts, it is challenging for isolated interventions to move the needle on school achievement measures. In a recent study that analyzed intervention effects across 141 large-scale RCTs, the average effect size on achievement was .06 SDs, with only 23% of the effects being significantly greater than zero (Lortie-Forgues & Inglis, 2019). For RCTs in general, median effect sizes on standardized tests in reading and math tend to be small to medium in size (.04 to .09 SD), making differences hard to detect unless sample sizes are very large (Kraft, 2020). In the case of the many individualized programs used in today’s classrooms to supplement core curricula (typically only for an hour or two each week), the challenges of demonstrating measurable effects are substantially greater.
Are RCTs more or less likely than QEDs to show treatment effects? In reviewing 645 experimental studies in math, reading, and science, Cheung and Slavin (2016) reported a significant advantage in the effect sizes obtained in QEDs (M = +0.25) over RCTs (M = +0.16). Similarly, Pellegrini et al. (2021) found that effect sizes in elementary math studies were a significant 0.12 SDs higher in QED than RCT studies. However, two other recent reviews, one focusing on secondary reading (Baye et al., 2019) and the other on struggling readers (Neitzel et al., 2022), revealed only small, nonsignificant differences favoring QEDs. Supporters of RCTs rightly argue that QEDs are more susceptible to biased sampling, due perhaps to more motivated or progressive schools choosing the innovative program over the current one. On the other hand, supporters of QEDs can fairly argue that schools’ higher motivation to implement the innovation with fidelity should naturally occur when they select it out of need and interest
Applied educational research faces many uncertainties and complexities. Accordingly, I recommend that program developers and researchers attend carefully to the conditions that impact the ability and motivation of participating schools to implement the target intervention in a quality and realistic way. Where RCTs seem likely to foster such conditions, striving for Tier 1 evidence and the gold standard makes perfect sense. But where RCTs seem likely to deter promising school adopters from participating in a study or weaken program usage, a QED can offer a valuable “silver” lining.
References
Baye, A., Inns, A., Lake, C., & Slavin, R. E. (2019). A synthesis of quantitative research on reading programs for secondary students. Reading Research Quarterly, 54(2), 133–166. https://doi.org/10.1002/rrq.229
Cheung, A.C.K., & Slavin, R.E. (2015). How methodological features affect effect sizes in education. Educational Researcher, 45(5), 283-292.
Every Student Succeeds Act (2015), S.1177—114th Congress. Retrieved from https://www.gpo.gov/fdsys/pkg/BILLS-114s1177enr/pdf/BILLS-114s1177enr.pdf.
Kraft, M. A. (2020). Interpreting effect sizes of educational interventions. Educational Researcher, 49 (4), 241–253
Lortie-Forgues, H., & Inglis, M. (2019). Rigorous large-scale educational RCTs are often uninformative: Should we be concerned? Educational Researcher, 48(3), 158-166. doi:10.3102/0013189X19832850
Morrison, J. R., Ross, S. M., & Cheung, A. C. (2019). From the market to the classroom: how ed-tech products are procured by school districts interacting with vendors. Educational Technology Research and Development, 67(2), 389-421. doi: 10.1007/s11423-019-09649-4
Neitzel, A. J., Lake, C., Pellegrini, M., & Slavin, R. E. (2022). A synthesis of quantitative research on programs for struggling readers in elementary schools. Reading Research Quarterly, 57(1), 149–179. https://doi.org/10.1002/rrq.379
Pellegrini, M., Lake, C., Neitzel, A., & Slavin, R. E. (2021). Effective programs in elementary mathematics: A meta-analysis. AERA Open, 7(1), 1–29. https://doi.org/10.1177/2332858420986211
U.S. Congress (2001) No Child Left Behind Act of 2001. Public Law 107-110. Washington, DC: Government Printing Office.
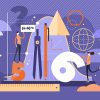